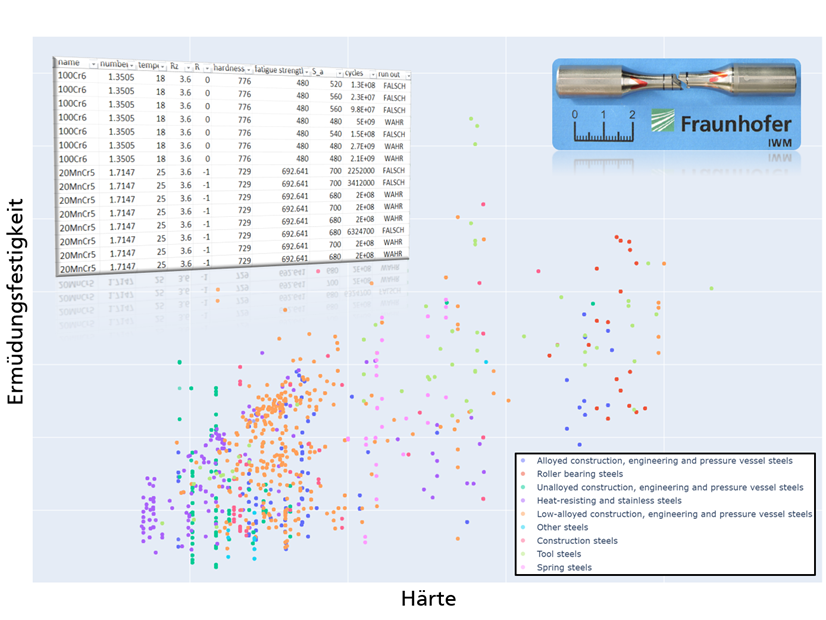
At Fraunhofer IWM, digital methods are developed which focus on a systematical use of material data for accelerated product development. Material data stored in structured data spaces and semantic knowledge graphs enable an interoperable connection to analyzation tools that cover the specific steps along the product development chain. In the frame of the internal Fraunhofer research project “UrWerk” such a data space has been developed for the “Fatigue assessment of high-strength steels” use case.
The life expectancy of components is influenced by a variety of parameters, including the metallurgical processing route, considerations concerning different hardness measurement methods, loading parameters in service and finally fracture surface characteristics under differing failure modes. Therefore, the change of parameters such as hardness and surface roughness as well as many others along each step of the process chain were tracked in a specific knowledge graph.